The Australian Institute of Health and Welfare (AIHW) has reported for the first time linkage and analysis of data by their Data Linkage Unit from the following six sources:
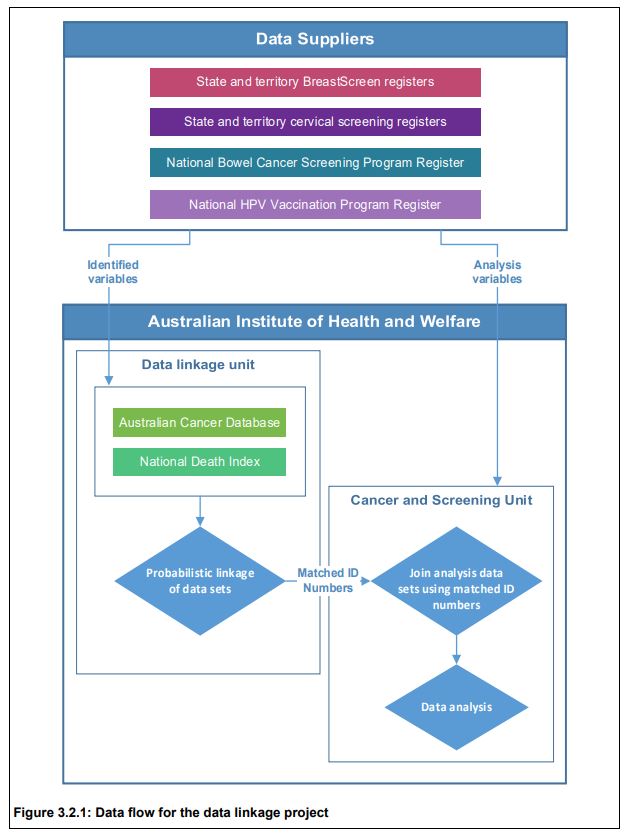
• BreastScreen Australia registers from the 8 states & territories;
• National Cervical Screening Program registers from each state/territory;
• the National Bowel Cancer Screening Program Register;
• the Australian Cancer Database;
• the National Death Index; and
• the National HPV (human papillomavirus) Vaccination Program Register.
Privacy was protected as no one person had access to both the identified data and analysis variables. Data linkage was carried out in a step-wise fashion using identifying variables names, sex, date of birth and postcode. Probabilistic data linkage based on the method developed by Fellegi and Sunter (1969) was used.
Retrospective cohort studies were undertaken for breast, cervical and bowel cancer to assess survival for screen-detected compared with non-screen-detected cancers.
Breast cancers were identified on the Australian Cancer Database by ICD-10 code (C50) with date of diagnosis between 1 January 2002 and 31 December 2012 inclusive, for women aged 50–69 at diagnosis. These were linked with available data from BreastScreen registers (from 1 January 2000), and the screening history prior to each cancer used to assign a screening status to each breast cancer. These were: screen-detected cancers; non-screen-detected cancers in screened women; interval cancers; non-screen-detected cancers in never-screened women. These individuals were then linked with data from the National Death Index to ascertain date of death and cause of death for those who had died by 31 December 2015.
Similar processes were undertaken for cervical and bowel cancers to create a National Screening Data Set. National identification numbers provided by the AIHW Data Linkage Unit to the AIHW Cancer and Screening Unit allowed the formation of a de-identified national cancer screening data set for analysis.
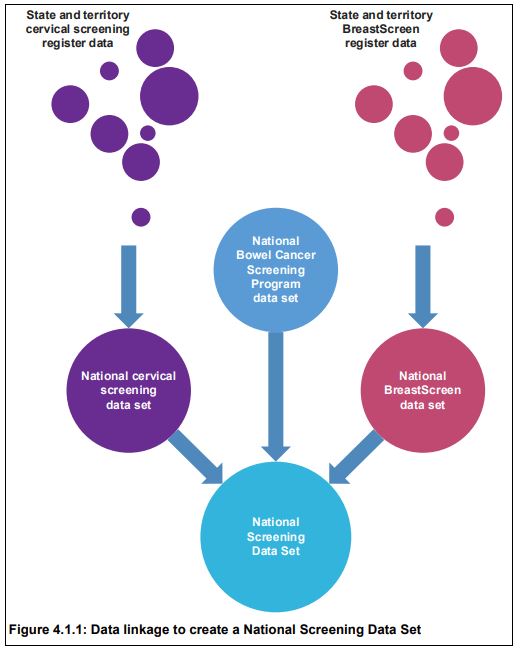
The Australian Cancer Database was linked to the National Screening Data Set using an outer join, meaning that all data from both data sets were retained in the final data set, which then comprised all screened individuals irrespective of whether they were diagnosed with a cancer, and all individuals diagnosed with a cancer irrespective of whether they were screened. This was important to ensure that breast, cervical and bowel cancers that were not detected through screening could contribute to the analyses, and that screening behaviour related to prior cancer diagnosis of any cancer type could be assessed.
Linkage with the National Death Index was the last step in the data linkage process. After linkage, there were 15,238,666 unique individuals in the project. The majority (61.7%) of individuals in the data linkage project had only one type of event (that is, appeared in only one of the data sources), while 24.6% experienced 2 event types, and 12.4% experienced 3 event types.
Three individuals experienced all 6 event types in the project, meaning that 3 individuals had a screening mammogram through BreastScreen, had a cervical cytology, histology or HPV test, were invited to the bowel screening program, were diagnosed with cancer, received at least 1 dose of HPV vaccine, and had died.
Breast cancer
There were 73,440 breast cancers diagnosed in the cohort selected for survival analyses (women aged 50–69 diagnosed 1 January 2002 to 31 December 2012). Women diagnosed with screen-detected breast cancers were less likely to die, and those who did die were less likely to die from breast cancer than women whose breast cancer was not screen-detected, with 50.5% of deaths in women with screen-detected breast cancer due to breast cancer, compared with 74.2% in never-screened women.
Cervical cancer
There were 6,897 cervical cancers diagnosed in the cohort selected for survival analyses (women aged 20–69 diagnosed 1 January 2002 to 31 December 2012). Women diagnosed with screen-detected cervical cancers were less likely to die, and those who did die were less likely to die from cervical cancer than women whose cervical cancer was not screen-detected, with 67.7% of deaths in women with screen-detected cervical cancer due to cervical cancer, compared with 78.7% in never-screened women.
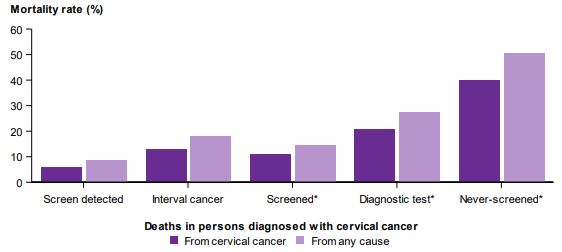
Never-screened women diagnosed with cervical cancer had the highest risk of cervical cancer mortality. The unadjusted hazard ratio for cervical cancer mortality for women diagnosed with cervical cancer was 0.11, i.e. 89% less chance of dying of cervical cancer than a never screened woman. All cause mortality was also statically significantly lower.
Bowel cancer
There were 31,427 bowel cancers diagnosed in the cohort selected for survival analyses (people aged 50–69 diagnosed 1 August 2006 to 31 December 2012). Of the diagnosed bowel cancers, 3,316 (10.6%) were screen-detected. The relatively low proportion is due to the need to be invited to have a bowel cancer detected through the screening program (previously limited to those turning 50, 55 or 65). The proportion of bowel cancers that are screen-detected is expected to increase with the introduction of biennial screening for all Australians aged 50–74 from the year 2019 (see previous post). People diagnosed with screen-detected bowel cancers were less likely to die, and those who did die were less likely to die from bowel cancer than people whose bowel cancer was not screen-detected, with 65.8% of deaths in people diagnosed with a screen-detected bowel cancer due to bowel cancer, compared with 78.1% in people never-invited to screen.
Further publications from the project are to follow. This first report clearly shows the value of data linkage, and overwhelmingly, the public health benefit of both Government subsidised screening programs and of participating in them!